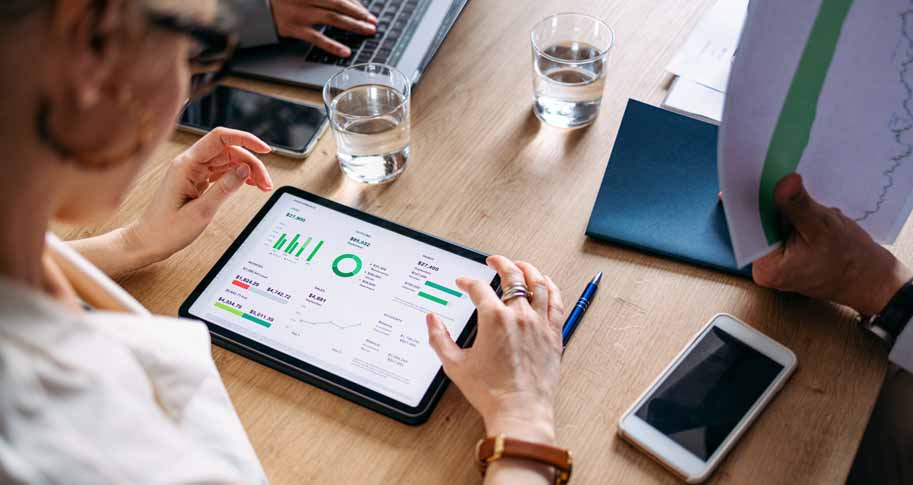
Experts have noted a lack of variance in the current methods researchers use to validate results, which increases the risk of allowing bias into their studies. Since business research often informs business strategy, the stakes are higher than you might expect. If research teams overlook severe distortions by not distinctly evaluating each measurement, the inaccurate data could lead managers to make incorrect conclusions and misguided actions.
Even the cleverest tactician errs when forming strategy based on unsound information. To better understand how errors from potential biasing factors affect measurements, Profs. Christopher Rosen, Paul Spector (USF), and Cheryl Gray (Montclair State), took a unique analytical approach in “Are Biasing Factors Idiosyncratic to Measures? A Comparison of Interpersonal Conflict, Organizational Constraints, and Workload.” The researchers considered measurements independently in their study rather than following conventional practices and assuming biasing factors would have an identical relationship with every measure that uses the same reporting method.
Biases in measures of workplace stress
Error is nearly impossible to avoid, but research teams must work to eliminate as much error as possible, ensuring their results accurately represent the situation they are studying. Bias occurs when researchers misclassify or incorrectly measure critical variables in a study, such as participant responses or collection methods. Often, locating biasing agents and separating them from other extraneous factors moves a team far closer to precision.
Bias can come from anywhere: participants, testing material, and researchers themselves all become potential culprits for introducing bias in a testing environment. Updating the challenging and inefficient techniques of finding factors responsible for bias and distortion is direly needed. Having the most effective way to identify these factors when measuring organizational variables for businesses is necessary because if firms cannot access accurate data concerning their organization, their managers are liable to make dangerous decisions.
What complicates these efforts is the simple fact that business operations involve numerous organizational variables. Spector, Gray, and Rosen, however, argue that experimenting with one would provide insight into others: workplace stress. Employees are the foundation of workplace culture and drive nearly every other organizational variable. Spector, Gray, and Rosen studied the Interpersonal Conflict at Work Scale (ICAWS), the Organizational Constraints Scale (OCS), and the Quantitative Workload Inventory (QWI), three popular measurements of stressors employees often encounter in a work environment. They selected these widely used scales because consultants often administer the three tests as a unit and the measures utilize a self-reporting response style. Observing the three measurements together allowed the researchers to witness the extent to which biasing factors affect each scale.
The researchers focused on recording the effects of five potential biasing factors: hostile attribution bias, negative affectivity (neuroticism), mood, neutral object satisfaction, and social desirability. These attributes are particularly relevant to the stressor scales, and while conceptually distinct, some elements like mood and negative affectivity tend to overlap in the field. Throughout three studies, the researchers recorded biases as they appeared while participants completed the three assessments. After observing how the different factors uniquely interacted with measures across their research, Spector, Gray, and Rosen provided evidence that research studies attain more accurate results by identifying and controlling for specific sources of method variance when measuring organizational variables.
Quality data
Business research – whether conducted by academia, think tanks, consultants, or individuals within the organization – often drives strategy and practice. Biased measurements, especially when evaluating organizational variables, give rise to distorted data that often translate into poor decisions by decision makers. Accurately measuring employee metrics like employee stress is crucial to obtaining quality data for a management team to reference while strategically planning.
By reworking our understanding of how biases affect measurements of organizational variables, researchers can protect people on various levels of a corporate ladder. For example, biases can negatively influence employee performance reviews. Evaluations originally created to highlight improvement and talent development now skew feedback, demotivating employees and managers in the process. What’s more, managers may gain an inaccurate picture of the climate and culture of their organization. By addressing biases within the measures themselves, experts can assist firm management teams in fostering healthier work environments and enhancing employee wellbeing.
Company leadership has a duty to build confidence in the company and to ensure that employees, customers, and investors alike trust their judgment. Measurement bias noticeably affects how stakeholders perceive a company. Misrepresented performance metrics or customer satisfaction ratings harm a firm’s reputation. Accurate data – from the number of customer service calls resolved successfully to net promoter scores – not only informs leaders on the state of the business and where it might be headed, but it also enables internal and external stakeholders to have faith in their leadership’s choices.
When assessing for error within organizational research, organizational scientists and practitioners can improve how effective firms are in locating biases. The research team countered the notion that biasing factors similarly affect measurements that share reporting methods. Spector, Gray, and Rosen advanced researchers’ ability to provide reliable data, however, by showing how biasing factors uniquely affect different measurements. As data quality improves, so will strategic analysis within your company. Researchers can now assure managers that their firms use accurate data to increase performance. By recognizing the potential sources of distortions, managers can nurture a positive organizational culture, maintaining stakeholder trust. Leaders can enhance their ability to steer their organizations toward sustainable success in a competitive and dynamic marketplace by embracing unbiased measurements.