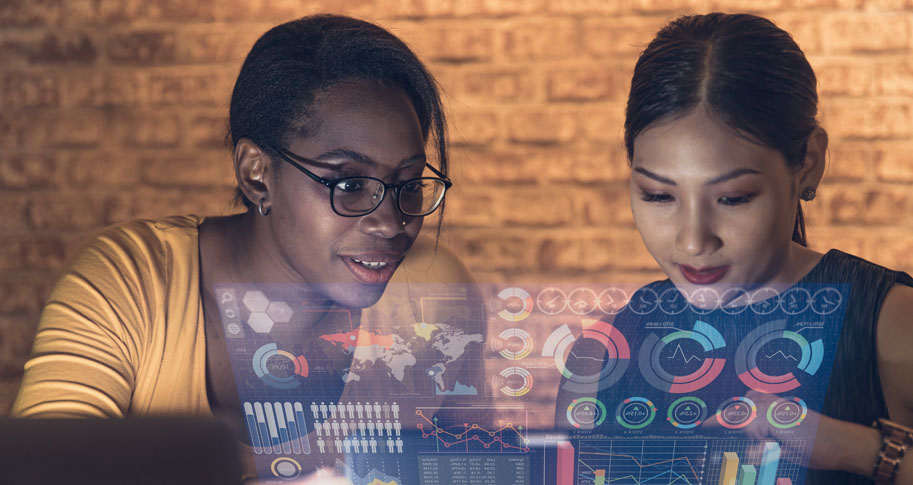
The world Is overflowing with data. Sports statistics, college enrollment numbers,
how many likes a tweet may receive. In the right hands, all this information has value.
Firms can use these numbers to their advantage, but who could possibly transform this
information into an asset? That’s where data scientists come into play.
What is a Data Scientist
Companies in increasingly varied sectors are using data scientists. Data scientists help corporations make informed decisions and run efficiently. They are essentially gatherers and interpreters of large sets of data. A CEO or
other executive will pose a question and a data scientist will use statistics, computer
science and mathematics to find a satisfactory answer. For example, a CEO might inquire
about the best way to invest product development funds, and it is the scientist’s
job to carve a path through the numbers.
A data scientist’s responsibilities break down into a simple order of operations.
Of course, the devil is in the details, but for simplicity’s sake we can dissect the
process using generalizations.
Initially, the researcher must understand their charge. In doing so, they perform interviews with stakeholders in order to
appreciate why the question was proposed by their company in the first place. By consulting
with those who have an interest in the company, a scientist may gain a new perspective
while analyzing data.
Data scientists might also research previous approaches other data scientists took to solving similar problems. By studying past examples, data scientists formulate
strategies for their own data collection and further analysis. Anything beneficial
a scientist learns about their data set streamlines the following process, saving
valuable time.
Once the scientist has fully assimilated their background knowledge, data collection
ensues. Relevant data comes from a variety of sources and appears in two forms. Data
can be either structured or unstructured.
Structured Data vs Unstructured Data
Structured data comes from sources that have a planned model of how it will be stored
and presented. Structured data is usually stored in relational databases: data storage
mechanisms that allow the user to implement storage, organization, and retrieval functions.
Collection methods vary from user surveys to browser cookies. This variety of data
is easily searchable and accessible.
Conversely, unstructured data is not arranged in a preset design. It cannot be stored
in relational databases because it can come in so many different forms. Unstructured
data can be emails, text files, social media posts or mobile communications. Data
attained through digital surveillance or satellite imagery could also be considered
unstructured.
A data scientist might also have to generate unstructured data themselves by performing
external customer surveys, internal employee surveys, holding interviews or scouring
social media for relevant information. Depending on where the scientist works, data
generation might be a heftier step. For example, a data scientist employed by a corporation
would have to create more data than one working for a government agency, where data
is more readily available. Scientists must investigate many distinct aspects of the
information they are trying to acquire to get the full story.
Making Valid Connections
Once the data scientist has gathered all the necessary and relevant data, they must
now validate it and find connections between their data and the original inquiry.
Data validation is the process by which a scientist guarantees the quality and accuracy
of the data they are using in their research and publication. A set of acceptable
values must be set during the validation phase of research.
Data is analyzed under two types of edits— “hard” and “soft”—according to the European Commission’s Methodology for data validation handbook. Hard edits are rules that must be fulfilled due to logic and mathematics. For example,
parents cannot be younger than their children and data cannot contain information
from postcodes that do not exist. Soft edits are used for numbers and figures that
seem suspiciously high or low and should be reviewed for accuracy.
By validating information, the researcher ensures their data is accurate, coherent,
accessible, clear and timely. After the data has been collected and validated, the
scientist can begin to draw connections within their collection of data.
Critically thinking about information is necessary during analysis. A good data scientist
would look at their data and start asking questions like why and how. They might look for disparities or biases and try to determine why these exist and
what the company could do differently to try to change them.
Communicating Solutions
After the scientist has developed a complete strategy for the company, they then must
communicate it to stakeholders. Researchers employ different strategies to accurately
convey their findings. Sometimes the way a data scientist presents their plan makes
all the difference. Some companies won’t end up using the insights provided due to
the data team’s inability to precisely explain their discoveries, and more importantly,
sell their ideas.
People with STEM degrees sometimes struggle to explain technical ideas and processes
to those in nontechnical roles, said Karl Schubert, associate director for the data science program at the University of Arkansas. To
combat this growing rift, students in the program at the UA spend a lot of time studying
case studies, working with real data, and learning how to write and present conclusions
clearly.
“Working with people to be able to communicate with nontechnical people is really
important,” Schubert said. “So, knowing that is one of the reasons why we concentrate
very early on, and [students] will give deep details of what they’re learning, and
they will learn how to explain that to other people. How to boil that down. We try
to eliminate the bad stigma people have.”
The UA data science program sets itself apart by incorporating different disciplines from different colleges in its required courses.
Often, data science degrees are comprised of solely mathematics, statistics and computer
science courses. The UA program includes courses from the College of Engineering,
the Fulbright College of Arts and Sciences and the Walton College of Business, enabling
students to choose from 10 specialty concentrations. Schubert said this approach was
crafted in response to what companies reported they needed from their scientists and
generally failed to see in graduates.
The University of Arkansas: Shaping Tomorrow’s Data Leaders
Everyone has a different idea of what data science is. Type the phrase, “What is a
data scientist,” into a search engine and you will find hundreds of differing answers.
Due to this inconsistent understanding, companies often find their scientists lacking
in one aspect or another. In 2020, Arkansas business leaders zeroed in on this issue
and requested Schubert and other administrators solve it.
“What makes our program unique, is, the big three corporations in [Northwest Arkansas]
came to us and asked us for this program,” said Lee Shoultz, UA data science program
manager.
The companies’ interest in the UA program is steadfast. Since its inception, representatives
from Walmart Inc., Tyson Foods Inc., and J.B. Hunt Transportation Services Inc., along
with assorted start-up firms from across the state, continue to work together in an
advisory council. The council guides the program so that graduates have the skills
needed in the specific industry they will begin working in.
Data science programs are popping up all over the country, but these programs are
usually reimagined computer science programs, Shoultz said. UA administrators have
created 18 new data science courses, to ensure that students graduate with every essential
quality needed for the whole process of working as a data scientist.
The program is young and still changing. Last year, Schubert asked students about
their experience in the program thus far and what they would like to see changed or
added in the future. Students used this opportunity and left many suggestions, said
Jonathan Ivey, a sophomore in the program.
“I think the faculty is very dedicated to making [the program] better,” Ivey said.
“I even noticed a significant difference between my first semester and my second semester
that things got a lot better and a lot cleaner. What I really like about Dr. Schubert
is that he is really adamant about getting complaints, reading and listening to all
of them.”
Since data science is such a dynamic field, students must spend time determining what
aspect of data science they would like to concentrate on, Ivey said. The UA program
utilizes guest speakers and professors from diverse backgrounds to expose freshman
to the many different paths their degree, and career, could take. Ivey chose to concentrate
on computational analytics since he enjoyed computer science.
Ivey interned with J.B. Hunt over the summer and said he felt well prepared for the
experience. Additionally, he received praise for his ability to communicate technical
concepts to people with little background in computer science. He thanked the holistic
nature of the UA data science program for his capability.
“It makes things more complicated for the administrators, and that's visible, but
I think it makes a big difference in the way that we approach things,” he said. “I think if you want to get a really well-rounded experience, the program is great
for that.”