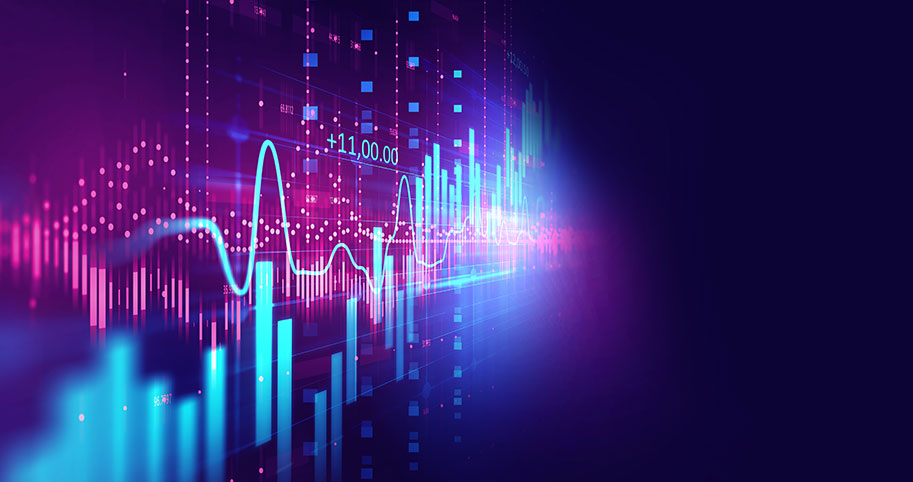
When ChatGPT emerged in late 2022, more people became aware of how their lives could be affected, for better or worse, by the use of generative AI. This type of artificial intelligence creates new content based on algorithms that analyze patterns and style. Because this type of artificial intelligence mimics human intelligence and language patterns, its advances make for splashier headlines regarding potential misuse. On the other end of the AI spectrum, generative AI’s quieter sibling, machine learning, is used to detect complex patterns in data, make inferences and select the best variables to predict certain outcomes. While not as flashy, machine learning can transform the way firms make decisions.
The accounting industry has embraced machine learning for its ability to analyze large datasets and detect fraud. In order to further understanding machine learning’s role in accounting, Walton College accounting professor Vernon J. Richardson, along with coauthors, Adam Booker (University of Denver), Victoria Chiu (SUNY Oswego), and Nathan Groff, conducted research on changes in technology that make machine learning easier and how these changes impact accounting research.
In “AIS research opportunities utilizing Machine Learning: From a Meta-Theory of accounting literature,” Richardson and his coauthors used Accounting Information Systems (AIS) and machine learning for specific research tasks, supervised and unsupervised models, and various research designs. The study’s primary goal was to align advances in machine learning with potential contributions to accounting research.
Machine Learning Technology Improves Efficiency
Machine learning allows computers to find patterns in data and use them to make predictions without being specifically programmed to do so. These algorithms enable a computer to imitate the way that humans learn and gradually improve its accuracy.
A machine learning algorithm reveals patterns in data by executing a process to solve an optimization problem, yielding a point (or set of points) with minimum error. This method provides insights beyond traditional statistical methods.
Machine learning has designed algorithms with the ability to drive cars, recognize spoken language, and find patterns in data. Therefore, these algorithms may help future researchers in detecting patterns in data, making conclusions, and selecting the best way to find certain outcomes.
Because machine learning allows for new study models, researchers can review the reliability of machine learning models. New technologies can change information sharing, examine text, find information not available in standard datasets and study large pieces of information.
In accounting, machine learning powers financial statement fraud detection, correction of accounting misstatements, classifying restatements, differentiation of bankrupt firms from non-bankrupt firms, and the identification of irregularities. Yes, humans can do these tasks, but they take time and are repetitive. Computers, however, thrive in carrying out repetitive tasks and thus allow for more exact estimates, even if they still have some limits relative to human judgment.
Machine Learning’s Effects on Accounting Research
A goal of this research was to provide a new framework to analyze and organize model selection and design to improve machine learning models’ effectiveness when performing tedious accounting tasks. The researchers found that machine learning can help accounting research tasks when grouped into prediction, classification, or clustering tasks. These approaches offer a road map for researchers looking to incorporate machine learning into their research as well as provides some insight into how firms could use these tools and models.
Prediction tasks, as the name indicates, predict the likelihood of specific outcomes based on outputs from machine learning models trained on past data sets. Machine learning has helped improve prediction of financial statement fraud and earnings forecasts.
In the prediction task, researchers used supervised machine learning algorithms to predict probability of financial statement fraud. The findings suggest that using mathematical models can provide better results on account of how human limitations exist for auditors when analyzing and testing large financial datasets. Researchers found machine learning models can provide early warning signs and identify companies with accounting misstatements more accurately.
Next, classification tasks use algorithms to give labels or tags to specific classes based on features and patterns. Machine learning helped classify companies based on bankruptcy risk, risk of misstatements and textual features in statements.
One model classified the probability of internal weaknesses in companies and outperformed manual classification methods. It was an effective alternative and a stronger predictor of restatements such as those for fraud and Securities and Exchange Commission investigations.
Lastly, clustering tasks group data points that have similar traits and find what relationships they have. Clustering has been used for identifying accounting irregularities because it captures firms’ similarity in financial ratios and groups them by finance and industry.
The study used financial reporting to cluster a large set of firms in different sectors, confirming that it can process a large dataset of different data types. Clustering analysis is a supportive tool for nonprofit and government data specifically. Different clustering methods can find better insights and understanding in different sets of data. It could be a useful tool for risk assessment, audit procedures or credit card data.
Machine Learning’s Effect on Everyday Accounting
Machine learning technology is changing the accounting industry as well as accounting research. Accounting obviously involves transactions between entities in an economic system, but blockchain technology allows for stakeholders to have a common database. This makes accounting more efficient and accurate and allows for all parties to stay informed of every process.
Blockchains allow for consistency in reporting formats, making restoration systems less costly for accountants and auditors. The combination of blockchain and machine learning allows auditors to save time because of the lack of a need for a public ledger.
On the other hand, the spread of information has become less centralized due to internet technologies, which have given a platform to anyone wishing to share information. Machine learning can also be used to examine these dynamics of public online communications. For example, it can examine online information flows and map the spread of topics. Prior research has shown that companies using social media to share corporate information attracted investors’ attention. However, researchers need more information about what leads to more efficient markets.
The Future of Accounting
Machine learning impacts firms’ innovation strategies and efforts to improve operations. It is expected to reshape the accounting field by transforming routine accounting processes, recognizing and understanding data patterns, enhancing the usefulness of textual data and visual information, and assisting the decision-making process.
Much of the accounting community is concerned that the automation will lead to a displacement of accounting labor, but the successful implementation of these technologies frees employees from boring and repetitive tasks and allows them to perform higher-order tasks. As we have discussed previously in Insights, process improvement does not always mean a headcount reduction.
Firms must first consider the research tasks and determine if machine learning can support it. Because firms are often more focused on clients rather than the practice as a whole, researchers examining practice can provide insights not apparent to many practitioners. As Richardson and his coauthors show, accounting has much to gain from leveraging machine learning, especially in big datasets.