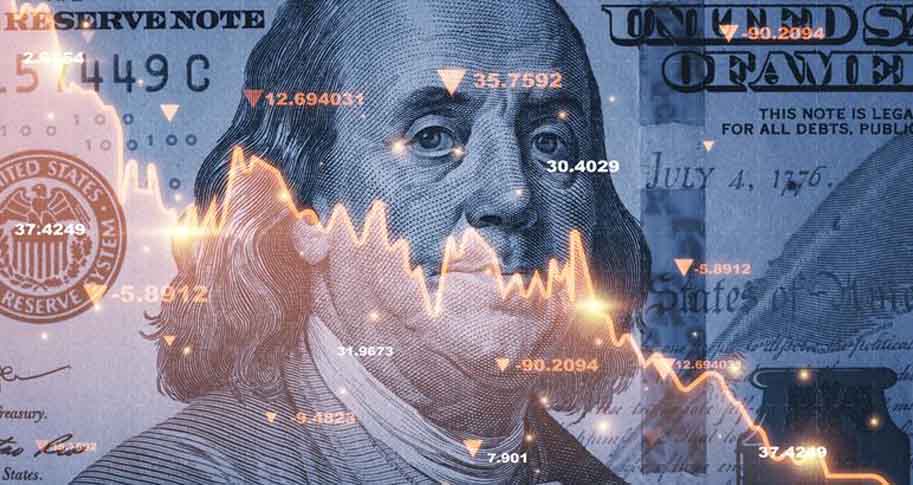
The idea that anyone can move up in the world isn’t unique to the United States, but it’s particularly cemented in the concept known as the “American Dream.”
That dream, historically speaking, represents something far more than materialistic success, but it is commonly associated with the opportunity for upward social mobility. Through “hard work, determination, and initiative,” as the Oxford English Dictionary puts it, Americans have the opportunity to achieve “success and prosperity.”
Many of us can see evidence of this dream, if not in the lives of those we know then certainly in books, television shows, and movies. But research indicates the socioeconomic situations of most Americans actually hasn’t improved much since the 1970s. In other words, social mobility in America has stalled. And a desire to understand why that’s the case, and therefore what might be done about it, was at the heart of a recent data analytics project by University of Arkansas graduate student Paul Gramling.
“The broad topic is just trying to uncover some of the general trends in why people are more likely to remain in the same socioeconomic status as their parents,” Gramling said. “I wanted to understand some of the factors at play and how a person’s future outcomes were shaped by their parents’ and their grandparents’ characteristics.”
Gramling took on the research as a capstone project in his Economic Analytics II class, part of the 10-month Master of Science in Economic Analytics program at the Sam M. Walton College of Business. But his personal interest in the topic goes back several years.
As a freshman at the UA, Gramling went home to Dallas during the pandemic. And in response to the global crisis, he helped a former high school teacher launch the Quispicanchi Project, a non-profit geared toward improving the socioeconomic conditions of families in a rural province of southern Peru.
Gramling, meanwhile, finished his undergraduate work with a degree in phycology and minors in international business, international economic development, global studies, and Spanish. When he learned about the master’s program in economic analytics, he saw it as a way to gain a foundation for the types of analytics and economic theory he wants to use in his professional career and with the nonprofit.
Gramling spent the winter break looking for data he could use for the capstone project in the spring, and diving into social mobility perfectly aligned with his interests. And the Panel Study of Income Dynamics, ongoing research on families that began in 1968, provided multigenerational data on topics like employment, income, health, education, and philanthropy.
“What I wanted to do with this project was consider both parents and grandparents and then also look at any differences between paternal and maternal lineages, because there’s very little about that in current literature,” Gramling said.
Because there was an overwhelming amount of information in the data, Gramling used machine learning and a variety of algorithms to help narrow his focus to trying to predict three outcomes: income from wages, self-reported health status, and education level. That still left more than 12,000 unique features as predictors for the outcomes, and machine learning, again, helped him identify patterns and sort through which ones were most important to predicting the different outcomes.
“The data processing part took about two-and-a-half months just to get my data set workable,” he said. “Then my actual analysis was like two weeks. So probably the biggest story from my project was how much more complex the data was compared to my classmates. I didn’t have as much time to really get into some of the specific results.”
The characteristics of parents generally have a bigger influence on someone’s social mobility than their grandparents, Gramling found, and that was no surprise he said since parents typically have more direct contact with their children than grandparents. He suspected there might be differences between paternal and maternal lineages but found that wasn’t the case.
“There really was no difference in the relative importance between variables coming from your dad’s side versus variables coming from your mom’s side,” he said. “They tended to have pretty similar, average importance across all three outcome variables.”
Figure 1: Density Plot of Combine Rank by Lineage
Figure 1 shows the similarity between your paternal (F) and maternal (M) lineages. Paternal and maternal plots are overlayed, thus creating the shaded region. Each point on the x-axis corresponds to a specific rank. The higher up the density plot goes; the greater number of variables at that ranking. Visual differences between the two lineage types exist at some rankings, where the blue and orange are seen clearly. However, these differences are statistically insignificant.
Gramling also wanted to identify which variable categories mattered most. Selected categories ranged from attitudes and behaviors to metrics such as income, wealth, and time usage. This allowed for a macro-level understanding of the types of characteristics coming from parents and grandparents that truly mattered.
Figure 2: Average Combine Rank of Variable Categories by Outcome
Figure 2 shows how different variable categories perform across feature reduction and impact estimation methods. A lower average combined ranking meant a category was more important for predicting one of the three outcome variables: Education Level (E), Health Status (H), and Income from Wages (W). Financial Distress, which has a very low average combined rank, tells us variables relating to parent or grandparent financial distress were very important for predicting a person's education level. This effect was consistently negative, as well.
With more time, Gramling said he would like to do more nuanced breakdowns within each outcome variable to see what the data might show. And even though he is finished with the class and about to graduate from the program, he said he plans to continue to explore the data in his free time.
The research is important, he said, because it can help parents understand how and why their attitudes and behaviors around things like money or their habits (like smoking) will influence future generations, which could lead them to make different, hopefully wiser, choices.
It also can help policymakers understand where to focus their efforts. For instance, Gramling found that if your parents or grandparents dealt with financial distress, then you are more likely to have a lower education level. Policymakers might take positive measures to increase access to capital for investments as a way to improve both the financial and educational fortunes of future generations. And they might work to counteract negative variables, as well.
As Gramling moves forward toward a professional career, he hopes other researchers, including future students in the program, will continue to mine the data set for insights.
“There’s just a myriad of different questions they could try and answer,” he said.
For his part, Gramling is grateful for the opportunity to answer a few of the questions during his time in the program.
“In 10 months,” he said, “I’ve probably learned more than I did in my entire undergraduate years. You get exposed to so many different aspects of not only economics but the analytical worlds. There’s a really good mix of different analytical techniques, which I appreciated. It wasn’t just the same thing over and over again. Then we dove into different disciplines within economics, so that was also super helpful. You just get such a broad view at economic theory and how to think like an economist, but then also got to analyze data, work with it and be comfortable with handling big data.”