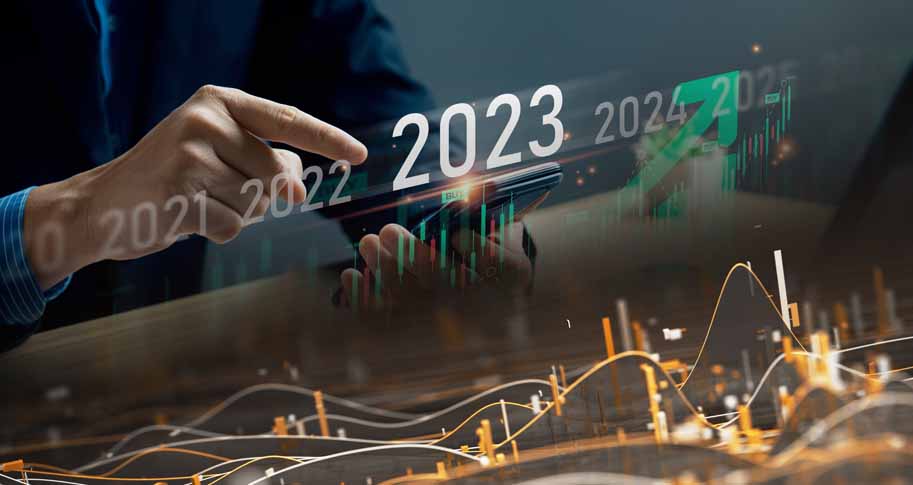
Automation drives two conflicting feelings. On the one hand, people are anxious about machines and artificial intelligence making their skills obsolete. Once stable, well-paying careers have evaporated, and there’s always talk about automating other positions to reduce costs by excising the costliest input: the human.
And now that generative AI tools create anxieties about threatening white-collar work, the fears about a humanless future of work have heightened. Automation is no longer merely academic for the policymakers and their children as it was when largely blue-collar households felt its effects.
On the other hand, automation and AI are just tools, and we need not turn to the dystopian when we consider our future with them. Rather than replace humans, they can augment and support our strengths as any tool does.
John Aloysius, professor of logistics at the University of Arkansas, helps us reconsider the integration of humans and machines in a positive light. He and his coauthors, Arkansas alumna Rebekah Brau (BYU), and Enno Siemsen (Wisconsin) describe and test a technique they coined, Human-Guided Learning, in their recent article, “Demand Planning for the Digital Supply Chain: How to Integrate Human Judgement and Predictive Analysis.”
They argue that human judgment is essential for forecasting even as modeling continues to make huge improvements. Demand planning needs human input, but the machine can greatly improve the response time of the human evaluator. The researchers’ new approach, Human-Guided Learning, would balance what humans excel at with the benefits of a machine. In essence, the human operator indicates special events, such as store promotions or upcoming holiday induced demands, that machines cannot readily incorporate into their forecasts.
For their research, Aloysius, Brau, and Siemsen worked with a partner firm and sifted through over 3 million events to test their new technique. They found that Human-Guided Learning was often one of, if not the, most accurate system for evaluating forecasts. Their work suggests the importance of the proper information integration system since accuracy can be case dependent.
Process of Forecasting
Aloysius, Brau, and Siemsen outline forecasting in the following way:
- Identifying systematic variation in past data
- Separating such variation from noise in the data
- Determining the stability of that variation
- And, finally, incorporating any unsystematic variation
Their intervention suggests that models excel at the first three steps, while human judgment is best at the final step. Therefore, a forecasting technique that uses automated tools to work with the systematic variation in the data to free up human creativity to work with unsystematic data variation should be more efficient, leading to better forecasting. The researchers’ novel approach, Human-Guided Learning, does just that. The human operator identifies special events that the machine would not be able to otherwise integrate, which produces better predictions for adjustments.
Aloysius, Brau, and Siemsen found specifically that Human-Guided Learning, while not the most accurate method of forecasting, performed incredibly well despite limiting what forecasters could input into the system. The authors also tested a method called Integrative Judgment Learning, whereby the forecaster estimates the strength of a signal and lets the algorithm take over from there. But Human-Guided Learning, which only notifies the system of an event and lets the machine estimate the strength of the signal as well, was the most promising. The potential value Human-Guided Learning could provide to firms implementing machine learning systems could be very high.
The Future of Demand Forecasting
Aloysius, Brau, and Siemsen argue for a hierarchy of integration methods, especially since Integrative Judgement Learning and Human-Guided Learning show clear advantages over other algorithm-based forecasting methods. These methods ask the forecaster to share their information with the algorithm and let it take over from there, so they reduce the effects of human bias.
More importantly for practitioners, the researchers conducted successful study using field data with a commercial collaborator, which suggests that their findings are already robust and applicable to real-world application. They offer a powerful tool that allows human forecasters to center the value they specifically bring to the table. After all, a tool is only as good as its operator. The researchers are currently taking the human guided machine learning approach to develop a tool that can be easily used by firms.
Aloysius’s research reminds us that the future of automation doesn’t necessarily need to be zero-sum. These tools can help us work better in the same way levers make it easier to move large amounts of weight or motors help us move faster.