Is Data Science a Fleeting Fad?
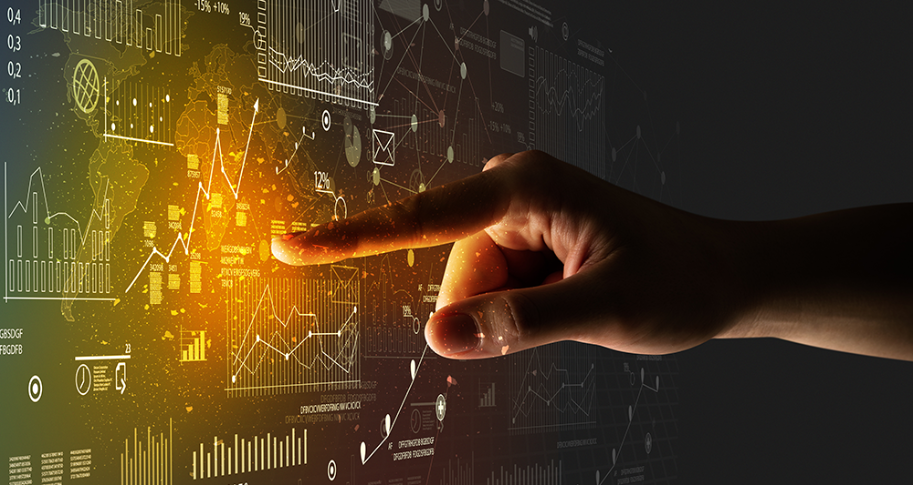
January 23, 2020 | By Matt Waller
Mid-way through our tenure as co-editors of the Journal of Business Logistics, Stan Fawcett and I challenged professors in supply chain management to conduct and publish more research around what we called DPB -- the confluence of data science, predictive analytics and big data.
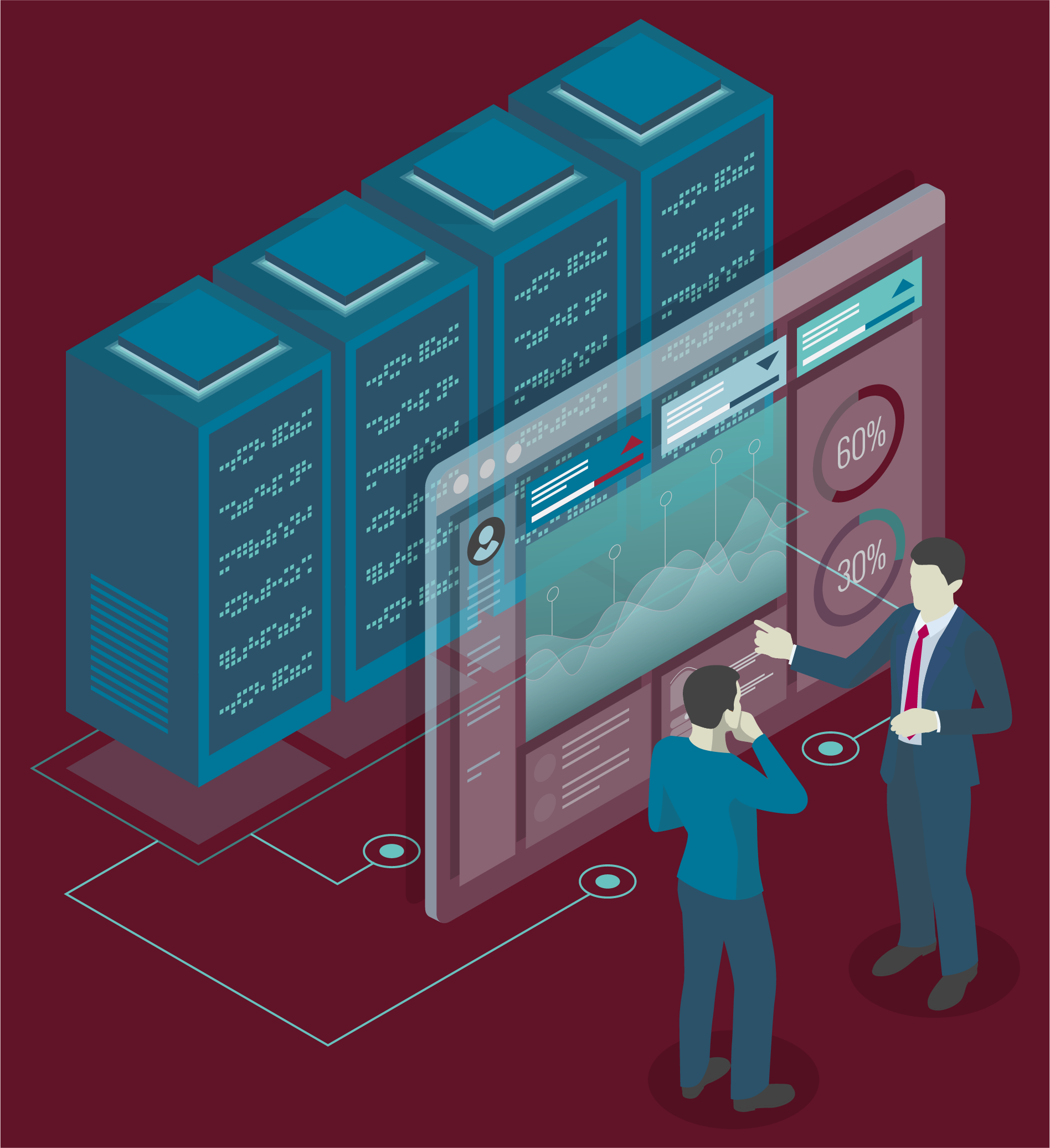
The reason was simple: The trends in those three areas were colliding in ways that would profoundly disrupt the logistics and supply chain industry. Therefore, academics needed to play their role in sorting through both the opportunities and the challenges in what was increasingly becoming a complex and overwhelming sea of information.
That was in 2013, and the need for more and better research hasn’t diminished. If anything, it has grown right along with the increasing amounts of data that’s available regarding supply chain decisions. What has changed over the last seven years, however, is the interest levels in data science and the measurable impacts DPB has had on the supply chain landscape.
The Growing Interest in Data Science
When we co-authored “Data Science, Predictive Analytics, and Big Data: A Revolution that Will Transform Supply Chain Design and Management” in the Journal of Business Logistics (Vol. 34[2]), interest in data science was low compared to today’s levels of interest. Google Trends, for instance, reveals a rapid rise in the relative number of searches for “data science” from 2013 until today.
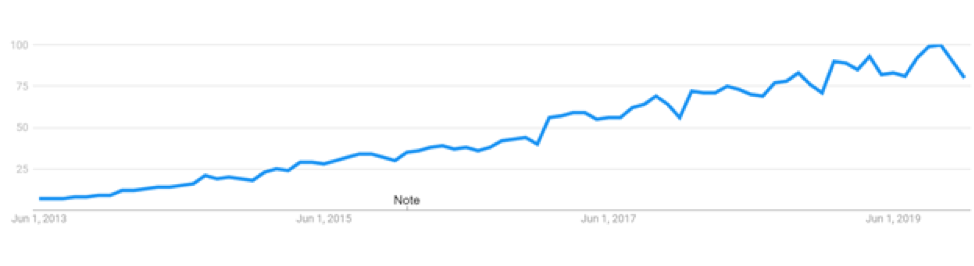
Researchers in logistics and supply chain, of course, are among those who have shown an increased interest in exploring the implications of access to so much data. The article we wrote, for instance, has been cited more than 855 times, according to Google Scholar. And it has been downloaded from the journal repository SSRN more than 3,500 times.
How Data Science, Predictive Analytics, and Big Data Impact Supply Chain Management
We believed then that data science, predictive analytics and big data would have a significant impact on supply chain management, but now it is clear that the impact has exceeded our optimistic expectations. Dozens of companies have been started in supply chain management around models that involve DPB, including a long list of startups developed in partnerships with supply chain accelerator component of Plug and Play. And many existing supply chain companies have added or expanded their use of DPB as a competitive advantage.
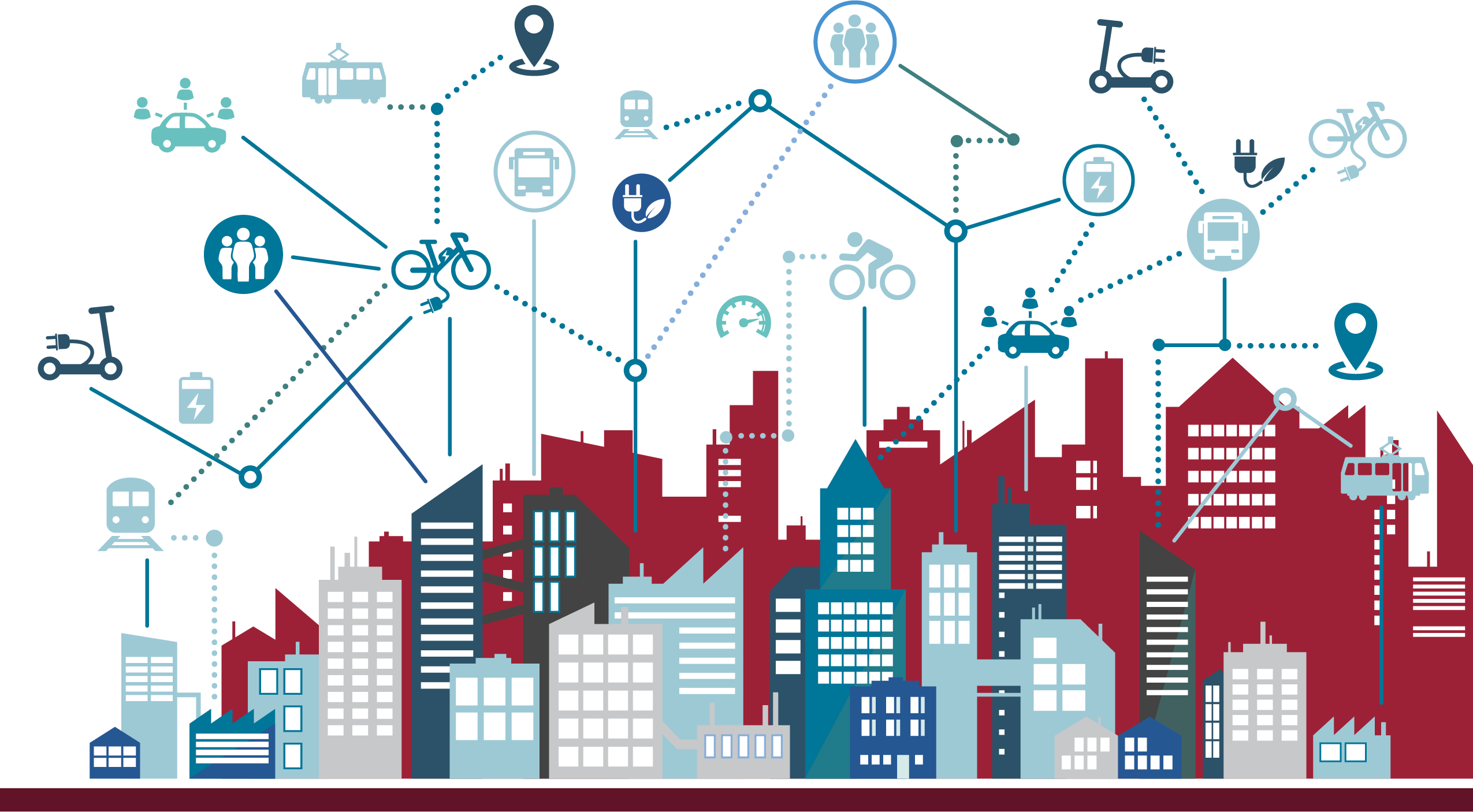
In our original article, we outlined areas we thought were obvious opportunities for application of data science in supply chain management. Now we can populate that outline with examples of how it has come to fruition (Table 1) in specific companies.
Table 1: Examples of potential applications of big data in logistics (this is a modified version of Table 4 in the original article).
User | Forecasting | Inventory Management | Transportation Management | Human Resources |
Carrier | Application: Time of delivery, factoring in weather,` driver characteristics, time of day and
date. Example: Wikenbach’s Lab |
Application: Real-time capacity availability. Example: JB Hunt 360 |
Application: Optimal routing, taking into account weather, traffic congestion, and driver characteristics.
Example: Carto's Traffico |
Application: Reduction of driver turnover, driver assignment, using sentiment data analysis. Example: WorkHound |
Manufacturer | Application: Early response to extremely negative or positive customer sentiment. Example: Thematic |
Application: Reduction in shrink, ECR, quick response, VMI. Example: Bakertilly | Application: Improved notification of delivery time, and availability; surveillance data for improved
yard management. Example: Veridian |
Application: More effective monitoring of productivity; medical sensors for safety of labor in
factories. Example: Atollogy |
Retailer | Application: Customer sentiment data and use of mobile devices in stores. Example: Nudge |
Application: Improvement in perpetual inventory system accuracy. Example: Daisy |
Application: Linking local traffic congestion and weather to store traffic. Example: Traf-sys |
Application: Reduction in labor due to reduction in misplaced inventory. Example: Apptricity |
Here’s a closer look at how those companies are using DPB to change logistics and supply chain for the better.
- Wikenbach’s Lab uses data analytics to process data about delivery routes. The data is collected by telemetry devices like GPS sensors and combined with real-time traffic data and weather conditions to help optimize last-mile deliveries.
- J.B. Hunt 360 Marketplace provides visibility to an inventory of more than a million trucks, the vast majority of which are not owned by J.B. Hunt.
- Carto’s Traffico uses crowdsourced information and other data to optimize delivery routes.
- WorkHound, a Chattanooga-based startup, analyzes feedback from drivers and translates it into insights that helps fleets retain their workforce.
- Thematic provides timely responses to customer feedback (positive or negative).
- Bakertilly provides a big data dashboard for manufacturing.
- Veridian uses big data in yard management.
- Atollogy uses big data for improving manufacturing productivity.
- Nudge Rewards uses smartphones to improve customer experiences in retail stores.
- Daisy Intelligence uses big data and AI to improve inventory management.
- Traf-sys has a number of shopper counting capabilities and applications.
- Apptricity provides solutions for inventory tracking.
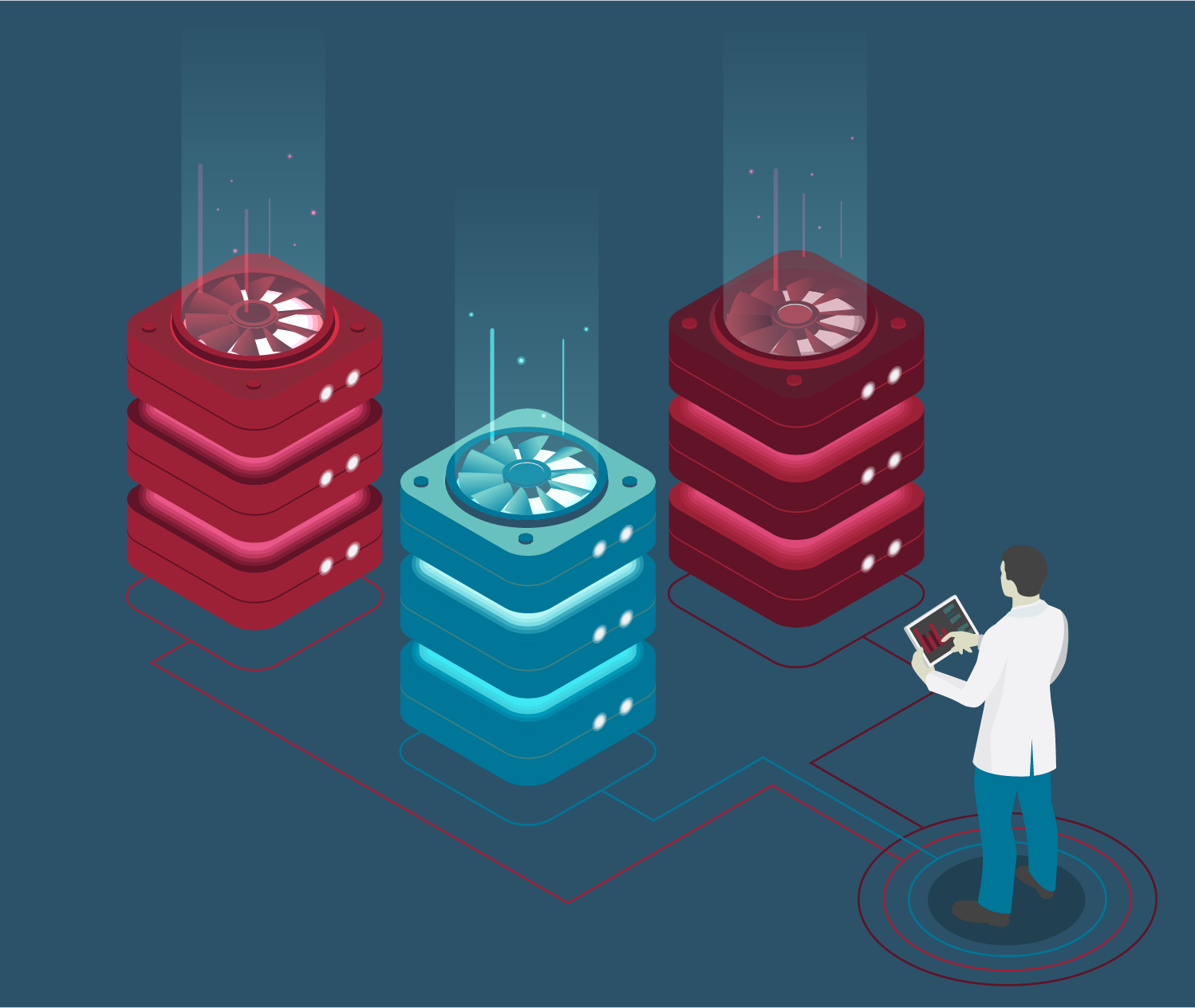
Theoretically grounded research plays a critical role in the development of technologies like the ones used by these and other companies. By applying quantitative and qualitative methods from a variety of disciplines and combining that with relevant supply chain management theory, we can propose solutions to pressing supply chain problems, predict outcomes, create workable frameworks, mitigate risks, and seize more and more opportunities. In other words, we can ensure that data in the supply chain industry isn’t just big, it’s useful.