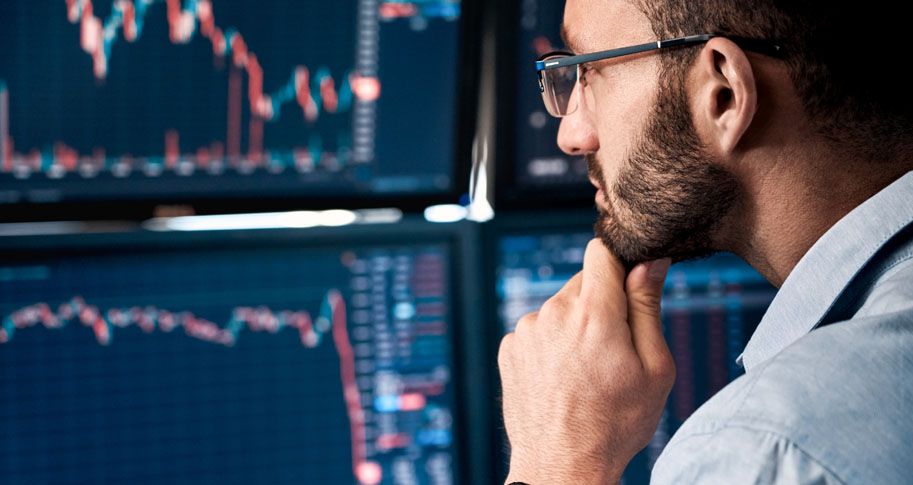
Should you buy, sell, or HODL? It’s not an easy question to answer even in the best of times. Investment always comes with some degree of risk, but so long as the investment isn’t betting on penny stocks or cryptocurrencies (or some strange mixture of both), earnings reports and historic performance must predict return to some degree, right?
Raji Raman, Ruba Aljafari, Viswanath Venkatesh, and University of Arkansas distinguished professor of accounting Vernon Richardson ask us, though, to consider the case of Facebook and Cambridge Analytica in 2018, one of Facebook’s many privacy scandals. The company’s stock price predictably tanked, and investors only cautiously continued to invest in the company. Relying on prior quantitative data would suggest a bullish projection for Facebook’s future, but regardless of those numbers, investor sentiment better reflected the bearishness of the situation. Indeed, following that sentiment saved many investors from losing money on Facebook during that downturn.
The authors do not discount those sources of quantitative information that investors have historically relied on. Instead, they see an unprecedented opportunity for the modern investor to make better decisions by mixing quantitative analysis with the analysis of underutilized qualitative information. Such analysis provides deeper, more nuanced information for investors and managers to act on.
The market is not a perfect optimization engine. It’s a human institution marked by our changing values and priorities. The authors argue that opinion mining can follow market sentiment. With the help of Ankur Arora, a PhD candidate at the University of Arkansas, the team of researchers developed novel Python and R programs to scrape and measure qualitative data on the market using online business journals.
The researchers found that the sentiment expressed by articles published in the Wall Street Journal between 2013 and 2016 predicted the abnormal returns during the same timeframe with a high degree of accuracy. Their program’s predictions were accurate even when traditional quantitative data suggested other outcomes.
Tracking Sentiment
The researchers assess the relative tone of a Journal piece by examining the author’s word choice. To systematically measure the tone of the pieces in their sample, the researchers use the Loughran-McDonald dictionary. The dictionary attaches a general sentimental valence to business vocabulary to aid researchers with textual analysis projects like this one. So, “overcharge” is marked as usually connoting something negative while “regulation,” which is often perceived as negative by businesspeople, is coded as merely litigious in nature.
The dictionary splits words into six categories – negative, positive, uncertainty, litigious, modal, and constraining – but Richardson, Raman, Aljafari, and Venkatesh were only interested in two for this study: positive and negative. The researchers had their program compare the Loughran-McDonald dictionary against the text of the sampled articles to compute the overall sentiment of the pieces. By analyzing the pieces’ sentiment collectively, the program could measure whether the Wall Street Journal’s coverage of a particular business on the S&P 500 was trending positive or negative.
In general, they found that positive sentiments correlated positively with cumulative abnormal returns, though they did observe diminishing returns at higher levels of sales growth. Likewise, negative sentiment correlated negatively with cumulative abnormal return. The data provides strong support for the observed “joint effect between sentiment from business journals and financial indicators.”
With proof supporting their hypothesis, the researchers trained an AI program to predict abnormal returns with their data set of S&P 500 companies. Once trained, their algorithm was 84% accurate when using both quantitative and text data, whereas quantitative data alone was only 70.7% accurate. Building on prior research that suggested the importance of sentiments in microblogs, tweets, and reviews for predicting market performance, the researchers argue these results “rigorously demonstrat[e] that sentiments…play an important role” in predicting returns.
Sentimentality and the Market
The authors’ work reminds us that money tells part of a larger story about how humans value and evaluate information. Market sentiment can certainly be positive and end poorly. Enron is certainly an instructive case. Years of positive sentiment did not stop people from suddenly souring on subprime lending in 2007. Tech companies certainly prove the inverse as well. For all the negative reporting on them that we can conjure, tech companies continue to post massive profits (with some hiccups).
These very human oversights are evident in the 15% gap between perfection and Richardson, Raman, Aljafari, and Venkatesh’s model, a careful antidote to any rising sentiment that data is omniscient – predictive algorithms do still live in the same neighborhood as clairvoyance.
I mention this because how not to use a tool is just as instructive as how to use a tool. The researchers point out that sentiment analysis is already in wide use, so they suggest that managers and investors should employ it as well when opinion mining is appropriate to forecast growth. Noticing positive sentiment can give managers the confidence to foster expansion, while negative sentiment can caution investors to reassess their adding a new stock to their portfolios, even when prior quarters only suggest a skyward trajectory.
In any case, the next time you’re evaluating apparently good-looking fundamentals, but you can’t quite dispel a negative gut reaction, consider Richardson and his coauthors’ suggestion: utilize diverse information sources and double check the sentiment.