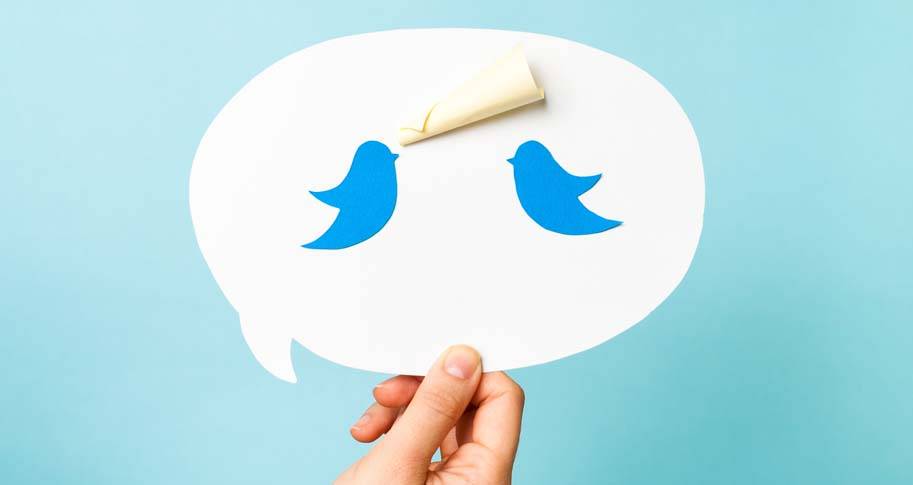
Note: This article is the first in a series on recent research conducted by Walton College students
Social media has engrained itself in our society. Politicians and voters are now able
to engage with each other like never before on websites like Twitter and Facebook.
Historically, voters have had to take politicians on their word and rely on journalists
to report on their legislative actions. Now, the public has a near-complete record
on what politicians say on the internet to their followers and how they act legislatively.
Furthermore, we can analyze the similarities and differences of their words and their
political actions, potentially holding policy makers to an even higher level of accountability.
University of Arkansas seniors Sai Elagandula and Griffin Fulton noticed how politicians were using Twitter to campaign and communicate to the American
public throughout recent election cycles. Elagandula and Fulton wanted to analyze
how senators’ tweets stacked up with their legislative decisions. To do so they trained
a classification model to track deviation between predicted political ideology based
on tweets and ideology based on legislative actions.
They used a political ideology tracker published by GovTrack, a website that enables users to track bills and members of Congress, as a basis
for comparison. From Jan. 3, 2019, through Jan. 3, 2020, GovTrack tracked 100 U.S.
senators and assigned each of them a score from most politically right to least according
to their legislative behavior. Topping GovTrack’s list for most politically right
was Marsha Blackburn (R – TN) with a score of 1.00; at the other end of the spectrum was Bernie Sanders (I – VT) with a score of 0.0.
Building the Right Data Set
To identify the deviations between online discourse and legislative action, Elagandula
and Fulton trained their model to categorize senators’ tweets as Democratic or Republican
using tweets from the Democratic and Republican party official Twitter accounts as
well as the chairs of the DNC and GOP as a standard for partisan affiliation. They
employed snscrape, an open-source social network scraper, to collect tweets from official party accounts
for the data set.
The DNC data set contained 6,752 tweets and the GOP set contained 6,166 tweets. Elagandula
and Fulton assigned each tweet the classification of Democrat or Republican accordingly.
They then used BERT (Bidirectional Encoder Representations from Transformers), a transformer-based machine learning technique for language processing pre-training
developed by Google, to turn tweets into encoded values. A percentage of words in
the tweets were replaced with a masked token, and BERT attempted to predict these
words. Elagandula and Fulton then sought to minimize the loss function by fine-tuning
weights and biases. In the end, they were able to achieve an accuracy rate of 93%.
They applied the model to tweets those senators made during their time in the 117th
Congress, from the start of the legislative session to April 20, 2022, and tracked
how many were categorized as the opposite party. There are only 86 senators ranked,
rather than the full 100, because tweets were collected from senators in the current
congress (117th) and the GovTrack rankings were made at the end of the previous congress
(116th). Senators not on either list were thus omitted. Using these statistics, they
then calculated a match rate to derive their own ranked list of predicted senator
political ideology based on tweets. Elagandula and Fulton then deployed a regression
analysis to see how twitter characteristics, margin of victory and years in office
to explain senators' match rate.
Fulton was especially intrigued by their regression analysis. The political ideology
match rate almost always correlated with their established explanatory variables.
They considered each politician’s average number of tweets per week, the number of
followers each politician had on their account, their margin victory in the most recent
election and the amount of time they had spent in office. They were fascinated by
the factors they discovered contributing to the perceived discrepancy between what
politicians tweet and the legislative actions they take.
When Elagandula and Fulton compared the list, they created with GovTrack’s rankings,
they found themselves surprised at some of the senators’ placement. Some politicians
were right on the money. For example, Senator Blackburn’s tweets were almost 100%
Republican, and Ted Cruz, who ranked No. 4 for most-right legislative behavior, rose
to No. 3 for his predicted ideology based on tweets. What is remarkable, however,
is how Senator Sanders' ranking climbed from most left-leaning, No. 86 for political
ideology based on legislation, to No. 61 based on his tweets. The researchers explained
this is largely due to his discourse on Twitter about domestic policy issues, which
are typically discussed by Republican Senators and traditionally resonate with the
right, according to Elagandula.
Trust the Tweets
This research may be encouraging for younger people. Roughly 37% of those who identify
as Democrat on Twitter are between the ages of 18 and 29, and 22% of Republican users
also fall in that demographic, according to a 2020 Pew Research Center study. As the world moves online, these numbers are growing, and political campaigning
has largely transitioned to targeted ads on social media. Twitter is currently the
main platform policy makers are using to communicate to voters, Elagandula and Fulton
said, which is why the public should be wary of what they read online when it pertains
to politics.
By tracking and recording what politicians say on Twitter, the public can better hold
policy makers accountable. The discrepancy between what politicians say and do can now be explained, or at least
monitored, by research using models and machine-based learning. Elagandula and Fulton’s project proves how accurate a text classification model can
be, especially when compared with GovTrack’s political ideology chart. However, the
range of political affiliation in the U.S. did create limiting factors.
Although Elagandula and Fulton had a short time frame and a narrow scope, they encourage
others to further train their model to identify ideological subgroups. Politicians
and their tweets could be considered alt-left or alt-right, but their current model,
which used the official party accounts as a foundation for categorizing tweets as
right or left, would not be able to identify them as such. The open-source, online
community has proven to be useful and many tools for creating machine-based learning
are free on the internet, according to Elagandula and Fulton. All of the tools they
used when creating their model were found for free on websites like GitHub or snscrape.
The senior’s project and methods can be viewed on their own GitHub portfolio. The accessibility of tools such as these speaks to the promise of continuing their
research or creating new projects.
If researchers monitor the words and actions of politicians, transparency, accountability and trust between the government and the public will
all increase naturally. They encourage Twitter users to take what they see on the platform with a grain
of salt and believe their project can be a helpful tool when deciding who to trust
and how to cast your ballot.
Connect with Walton College.
Stay Informed